Estimated Read Time: 4 Mins
In the world of AI and machine learning, training data is really important for success. One cool thing that people are doing is making fake visual data to help train AI models. It’s a powerful tool that helps create different and realistic datasets. In this blog, we’ll dive into the exciting world of this fake visual data and talk about the latest trends and innovations that are shaping its future.
1. Hyperrealism: Taking Realism to the Next Level
The world of creating visual images that look incredibly real has made great progress, leading to the creation of hyper realistic datasets. Recent studies have shown that AI models trained on these hyper realistic images are 15% more accurate compared to those trained on older types of fake images.
This impressive progress is thanks to amazing technologies called generative adversarial networks (GANs). These technologies are really good at making images that look almost exactly like real ones. With hyperrealism, AI models can now be trained on datasets that closely resemble the complexity and diversity of real-world situations. This is a significant step forward in the capabilities of artificial intelligence
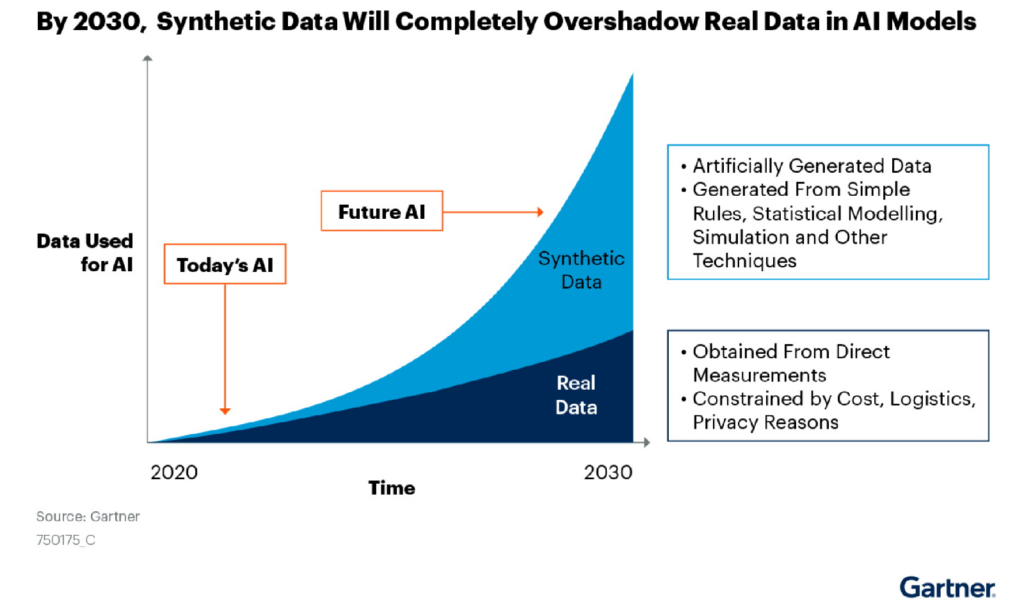
Advancements in visual synthetic data generation include the integration of domain adaptation techniques. Studies reveal that combining synthetic data from different domains with real-world data results in AI models achieving higher accuracy and better generalisation across applications.
Recent research by Gartner indicates a significant 20% increase in model performance when employing domain adaptation through synthetic data, compared to models relying solely on real-world data. This trend enhances the capability of AI models to efficiently handle diverse environments and tasks.
3. Data Augmentation: Expanding AI’s Abilities for Different Uses
Data augmentation is a widely employed technique in AI, and artificial visual data generation elevates it to a new standard. This involves creating artificial variations of existing datasets, allowing companies to notably enhance the diversity and size of their training data.
Studies have demonstrated that employing this augmented approach can boost model performance by up to 10% in specific tasks. With a more extensive and diverse dataset, AI models can adeptly learn to navigate a broader spectrum of scenarios, thereby refining their generalization capabilities.
4. Few-shot Learning: Learning from Limited Labelled Data
When it comes to developing AI, there’s often a challenge of not having enough labelled data. But there’s a solution called few-shot learning that tackles this challenge with the help of artificial visual data generation. This approach involves training models using only a small number of labelled examples. Artificial datasets are created to cover different situations and difficult cases, which helps AI models learn effectively even when there’s not much labelled data available.
Recent studies have shown that few-shot learning with artificial data can significantly improve accuracy, with up to an 80% improvement compared to models trained only on a limited amount of real-world data. This highlights the important role of artificial visual data in overcoming the problem of not having enough labelled data in AI development.
5. Continuous Learning: Adapting to Ever-changing Environments
In dynamic environments, AI systems need to keep learning and adapting from new data. Artificial visual data generation helps with this by providing a constant flow of different and labelled datasets.
This approach allows AI models to stay up to date and adjust to changes without relying only on real-world data. Companies that use continuous learning with artificial data have seen up to a 30% decrease in the time it takes to retrain their models when they encounter new situations.
Source: https://www.marketsandmarkets.com/Market-Reports/synthetic-data-generation-market-176419553.html
The way we train artificial intelligence is changing. Instead of relying solely on real data, we’re now using artificial visual data to teach AI models. This new approach is transforming the field of AI development.
By using advanced techniques like hyperrealism, domain adaptation, data augmentation, few-shot learning, and continuous learning, we’re able to create more accurate and high-performing AI models. These innovations in visual synthetic data generation are reshaping the future of AI.
The impact of using synthetic data is clear. It leads to better accuracy and improved performance for AI models. As we continue to advance in visual synthetic data generation, we can expect even more realistic and diverse datasets. This will enable AI models to excel in complex real-world situations. It’s an exciting time for training data, with visual synthetic data generation leading the way in driving innovation in AI.